On the misinterpretation of P>.05 in psychological research
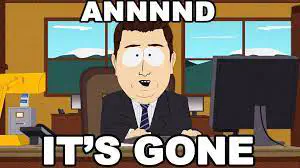
In psychological research, scientists often calculate ‘p-values’ to determine whether their hypotheses can be supported. P-values provide an insight into whether we can expect identified relations in a sample, for instance, whether men and women differ in how much self-control they have, or whether age is a predictor of wellbeing, are likely to also manifest or exist similarly in the broader population of interest who can’t be directly measured because it would be unfeasible to do so. Importantly, researchers often acknowledge in advance that they will only claim support for their hypotheses if the calculated p-value is below a threshold they set before analyzing their data; this threshold (which is usually set at .05) should generally be small enough that the researcher, if they were to (hypothetically) collect 100 separate samples to test the focal effects, would very infrequently (with a .05 threshold, only 5% of the time) find an effect when one doesn’t actually exist in the population. In other words, the p-value gives the researcher some confidence that they are not interpreting error variance as the effect. If the researcher finds the calculated p-value for the effect to be above their pre-set threshold, the ‘null hypothesis’ that there is no effect should not be rejected. In this instance the researcher should simply withhold judgement on any claim for an effect.
Lets provide a clear-cut example of this. Lets say I want test my hypothesis that age negatively associates with subjective wellbeing. I collect the necessary data from 5000 individuals varying across the age spectrum, analyze it, and calculate the p-value. If I had set my p-value threshold at .05, and then find the relation between age and subjective wellbeing has a p-value of .001 - below my threshold - I should reject the null hypothesis that age does not negatively associate with subjective wellbeing, and tentatively acknowledge support for the alternative hypothesis that age negatively associates with subjective wellbeing. If, rather, my calculated p-value is .24 - above my pre-set threshold - then I can’t reject the null hypothesis or claim support for any effect.
Unfortunately, evidence suggests the vast majority of psychological researchers do not get this right. Specifically, in cases when they find their calculated p-value to be above their pre-set threshold, they don’t simply withhold judgment on the alternative hypothesis, which is what they should do; rather, they explicitly or implicitly claim that the above-threshold p-value means the alternative hypothesis is incorrect, that there is absolutely no effect/relationship in the overarching population. Why are these researchers wrong if they interpret a nonsignificant effect as reflecting no population effect? Firstly, because the idea that there is absolutely no effect (e.g., no association between age and wellbeing) generally makes very little sense at the broader population level, particularly when using continuous data with an indefinite number of decimal places. In other words, an effect will pretty much always exist in the population of interest - all we need to do is understand its direction and magnitude. Tukey (1991) and Bakan (1966) make this clear:
“It is foolish to ask ‘Are the effects of A and B different?’ They are always different—for some decimal place” (Tukey, 1991, p.100).
“Why should any correlation coefficient be exactly .00 in the population? Why should we expect the ratio of males to females be exactly 50:50 in any population? Or why should different drugs have exactly the same effect on any population parameter? A glance at any set of statistics on total populations will quickly confirm the rarity of the null hypothesis in nature.” (Bakan, 1966, p.426)
Secondly, if the researchers instead had a vastly larger sample - say instead of having 500 participants to test their hypothesis, they had 1 million participants, they could guarantee that their (non-directional) hypothesis would be significant and thus supported. A high p-value thus generally indicates that one didn’t have enough data to find a significant effect and to therefore be able to say the identified effect was of a magnitude considered ‘strange’ if there was no effect at the population level, and is easily overcome by collecting more data that can identify even very small effects as strange amidst a true null effect.
What is the evidence indicating that psychologists make the stated mistake? Firstly, metascience research. Numerous metascience studies coded statements made within hundreds of psychology articles, and found between 38% and 72% of articles that reported a nonsignificant finding misinterpreted it as reflecting an effect absent in the population of interest. Also, I am personally involved in research that overcomes some methodological limitations of these metascience studies (e.g., our sample articles come are sourced from many more psychology journals than previous research), and my intuitive feel so far is that the problem may well be much worse (read, more prevalent) than previously reported. We’ll be submitting this research for publication in the coming months. However, a second way of substantiating our claim of this issue is simply to list here examples from recently published psychology articles. These statements below (we highlight in italicized bold where a nonsignificant effect is misinterpreted by the article authors as the effects absence) were very easy to find, and speaks somewhat to the scope of the issue.
“We found that when people are forming an impression, trait inferences are differently correlated across race and gender in stereotype-consistent ways. Thus, to the extent that physical strength and trustworthiness are negatively associated for Black men but unrelated for White men, sentencing decisions, which are influenced by how trustworthy a target appears, are more likely to be influenced by other attributes (e.g., physical strength) for Black than White male defendants.” (Xie et al, 2021, p. 1990)
“Across 17 studies, we found no evidence that choice causes an illusion of control. Choice rarely made people feel more likely to achieve preferable outcomes when all options were functionally identical, whether we used different outcome measures (Studies 1–3), made the process visual (Study 4), varied the levels of uncertainty (Studies 5–7), or increased the subjectivity of the outcome evaluations (Studies 8 and 9). Choice had such effects only when it conferred actual control (Studies 10 and 11). In the rare cases in which choosers felt more likely to achieve preferable outcomes (Studies 12–15), choice seemed to reflect people’s preexisting beliefs rather than cause an illusion (Studies 16 and 17)” (Klusowski et al., 2021, p. 170)
“The present study showed that although threatening and disgusting stimuli clearly impaired performance after they appeared (Experiments 1–3), participants did not inhibit them in advance. Rather, they were more alert when they were expecting any stimulus to appear: negative, neutral, or joyfulFurthermore, even though the interfering effects of threat and disgust were modulated by anxiety, these factors did not impact the preparation effect. Hence, these results imply that the preparation effect is a rigid mechanism that depends neither on the emotional valence of the stimulus nor on the anxiety level of the observer.” (Makovski et al., 2021, p. 263)
“Studies 3 and 4 examined causal links between couple identity clarity and commitment. In Study 3, people who recalled a time when they experienced low couple identity clarity reported lower commitment than did those who recalled an experience of high couple identity clarity or who completed a control prompt. Mediation analysis revealed that this effect was driven by decreases in couple identity clarity specifically and not by priming participants to write more negatively about their relationship or by decreasing their relationship satisfaction.” (Emery et al., 2021, p. 156)
Now it may be claimed that most psychological researchers don’t actually mean there is no effect when they communicate as such, and that they make ‘no effect’ statements only when the evidence suggests the effect is too small to be of concern (because if the effect is too small to matter, for communicative convenience we might as well declare it as not existing, right?). While we acknowledge this may characterize some psychology researchers, the above statements I’m afraid do not hint at this being the rule rather than the exception. Rather, these and other statements, in lieu of any other article text that provides clarification of what these nonsignificance statements really mean, communicate fairly clearly to the reader - particularly those outside of academia that may be less able to gather the authors real meaning - that their nonsignificant effect reflects the effects complete absence.
Also, a nonsignificant effect does not guarantee the population effect is smaller than expected - it may simply be that the researcher was ‘unfortunate’ with the sample they selected (i.e., their sample was not the best representation of the population - if they collected another sample, the effect may well be larger and more representative of the population effect), and that the true population effect is potentially much larger than expected. Finally, if we are to believe that researchers communicate nonsignificance as ‘no effect’ primarily because (they believe) it indicates the effect is minor in magnitude, one would also expect them to similarly employ ‘no effect’ statements when discussing significant effects that also are small in magnitude. However, this seems to be rarely the case. Rather, significant effects are nearly always considered important and thus ‘to exist’ in the broader population. This suggests the ‘no effect’ statements are primarily driven by whether or not the effect is significant, not the effects (likely) magnitude.
Is it problematic that psychological researchers generally make this communicative error of interpreting nonsignificance as an effect no existing? Absolutely. You wouldn’t believe the amount of money that goes into funding psychological research, much of it from the tax-payer that expects a fair bang for their buck. Thus, the least one can expect is that psychologists understand and communicate what their findings, and in this case nonsignificance, mean(s). Failure to do this breeds misunderstanding in those less methodologically or statistically savvy (e.g., the media) and/or those without the time or energy to scrutinize the study’s finer details, and adds weight to the growing feeling that, irrespective of what we’re told by governmental officials or others, we unfortunately can’t trust the science (see Stuart Richie’s substack for substantiation of this). An obvious solution to overcome this issue is for journal editors - the gatekeepers determining whether peer-reviewed research see’s the light of day - to get their act together and deal with this and similar issues, so that articles are only published that communicate their findings correctly (although admittedly, editors are time-poor as it is!). Other solutions could be to better ensure psychological researchers better understand the statistics they use, or to ensure statisticians are part of the research collaboration (which they generally are not) to keep them right.